01 Research
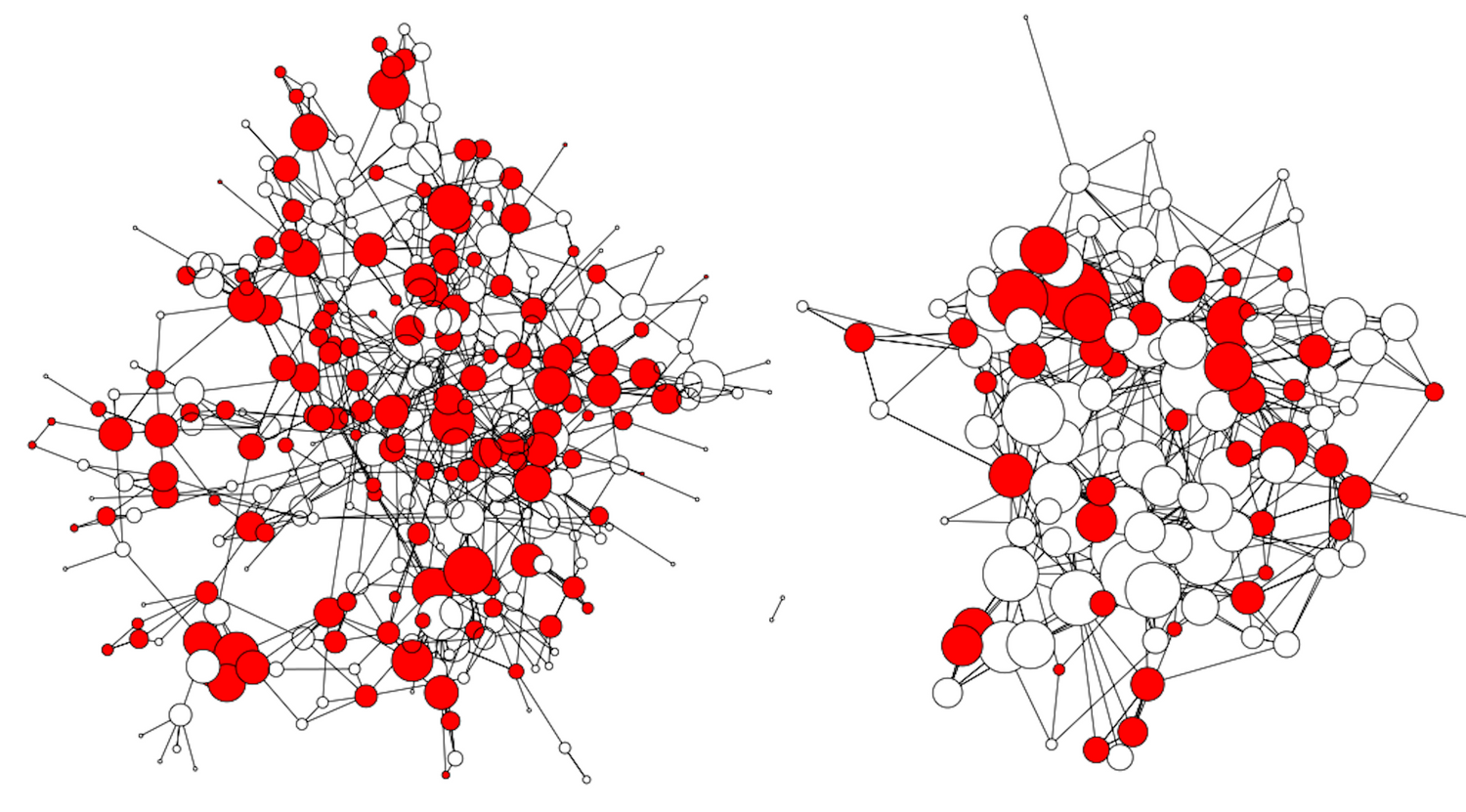
Collaborators: Nicholas A. Christakis (PI), Ilana Brito (PI), Francesco Beghini, Shivkumar Vishnempet, Drew Prinster, Eric Liu, Chengqi Xu
Although the human body contains as many bacteria as human cells, and there are about 200 times more bacterial genes than there are human genes, only a small number of bacterial species that colonize humans are known (perhaps < 5 percent) [1]. Little is known about the human microbiome because conditions necessary to grow the bacteria in the lab are difficult to reproduce. Recent advances in sequencing and the development of metagenomics make it possible to analyze the entire human microbiome at once. This involves sampling the microbiome and analyzing all of its DNA at once, taking advantage of supercomputing resources and advanced alignment algorithms to assemble genomes of previously unknown species and identify functional bacterial genes playing a role in normal human physiology and disease [2].
Previous studies suggest that the bacteria that live in our gut play an important role in the normal functioning of the human body, including maturation of the immune system; regulation of endocrine function and neurologic signaling; maintenance of bone density; modification of drugs; and elimination of exogenous toxins [3]. And, conversely, studies have identified a potential role of the microbiome not only in various bowel diseases, but also in atherosclerosis [4], obesity [5], diabetes [6-7], asthma [8-9], autoimmune disorders, and even in brain function and mental health conditions such as depression.
An important aspect of the human microbiome is that the bacteria that compose it have co-evolved together with human populations. Previous studies of the biological and sociological features of human social interactions suggest that natural selection has shaped social network structure and function [10-14]. While early research suggests that social relationships, especially within the household, are involved in gut microbiome transmission, the distribution of the human gut microbiome across social networks has not been studied [15-17]. Unlike our understanding of pathogens and the dynamics of infectious diseases, it is not known what role social transmission plays in the composition of the healthy microbiome. Furthermore, the link between the gut microbiome and non-infectious diseases described above implies that the spread of the gut microbiome across social networks may help explain epidemiology of obesity, cardiovascular disease, and other.
The proposed research is the first attempt to study the human gut microbiome metagenome across population-level networks of social interaction, examining the impact of the microbiome on community health. By focusing on a population in Central America, the study will also contribute to our understanding of the gut microbiome diversity in low and middle-income countries, as well as in Indigenous populations. The study aims to identify social highly-transmissible strains or mobile genes associated with either increased or decreased risk of disease, leading to better epidemiological models and novel therapeutic strategies.
Our hypothesis is that different gut bacterial species will cluster in distinct regions of social networks, and that these clusters will be associated with increased risk of elevated BMI, HbA1 and MAP, even after adjusting for known risk factors. Specifically, this would imply a statistically-significant correlation between topology measures of the village social networks and the same topology measures of co-occurrence networks of gut bacterial species and clades in the village.
Microbiome Networks
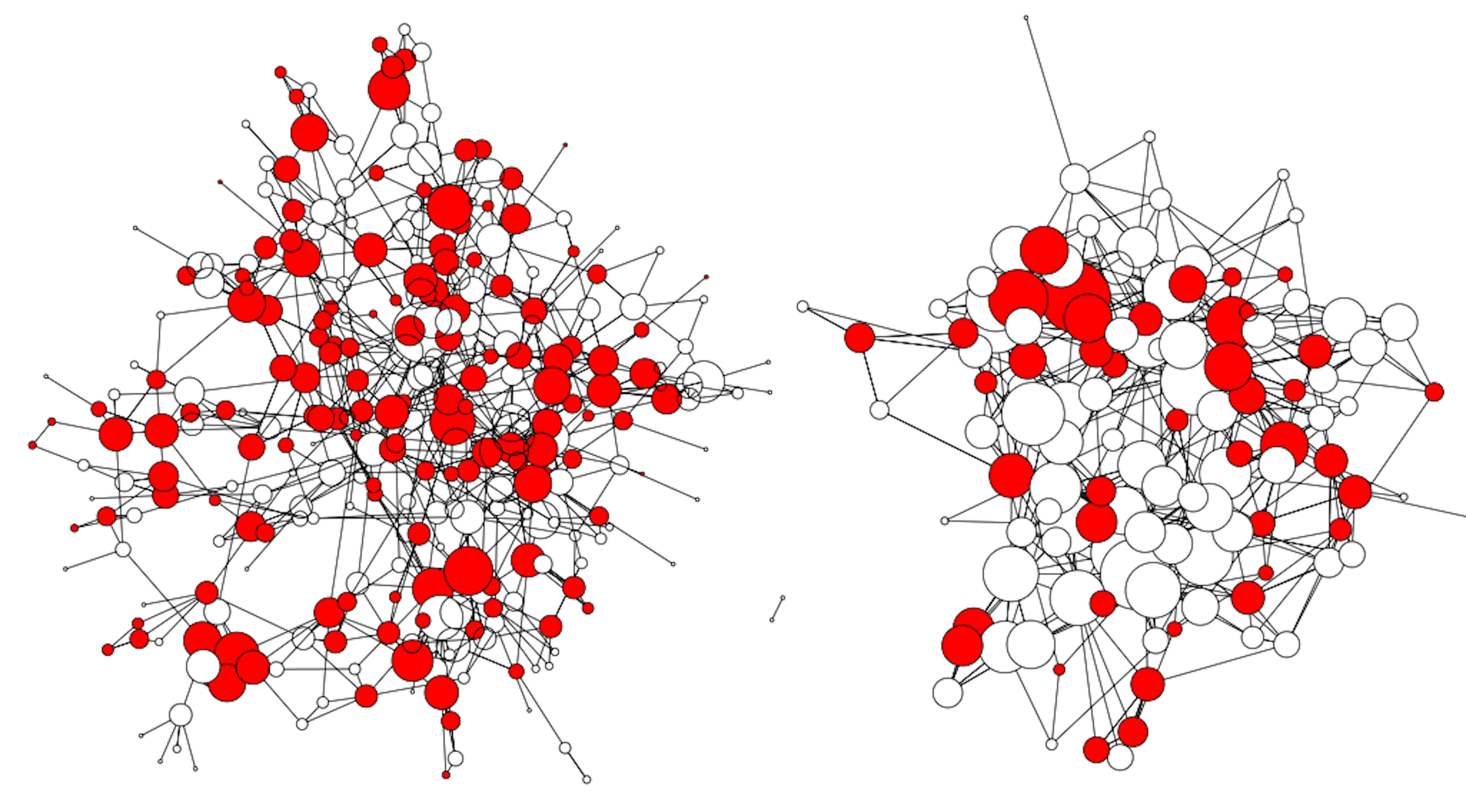
Distribution of respiratory disease across two village social networks in Honduras
Human Microbiome and Social Networks in Central America.
The purpose of the project is to describe the relationship
between social networks and the distribution of the gut microbiome in human communities.
We focus on a set of Honduran villages, where our lab has mapped
sustained face-to-face networks of interactions, sequenced gut microbiome
samples, and collected health measures and microbiome survey responses.
Collaborators: Nicholas A. Christakis (PI), Ilana Brito (PI), Francesco Beghini, Shivkumar Vishnempet, Drew Prinster, Eric Liu, Chengqi Xu
Although the human body contains as many bacteria as human cells, and there are about 200 times more bacterial genes than there are human genes, only a small number of bacterial species that colonize humans are known (perhaps < 5 percent) [1]. Little is known about the human microbiome because conditions necessary to grow the bacteria in the lab are difficult to reproduce. Recent advances in sequencing and the development of metagenomics make it possible to analyze the entire human microbiome at once. This involves sampling the microbiome and analyzing all of its DNA at once, taking advantage of supercomputing resources and advanced alignment algorithms to assemble genomes of previously unknown species and identify functional bacterial genes playing a role in normal human physiology and disease [2].
Previous studies suggest that the bacteria that live in our gut play an important role in the normal functioning of the human body, including maturation of the immune system; regulation of endocrine function and neurologic signaling; maintenance of bone density; modification of drugs; and elimination of exogenous toxins [3]. And, conversely, studies have identified a potential role of the microbiome not only in various bowel diseases, but also in atherosclerosis [4], obesity [5], diabetes [6-7], asthma [8-9], autoimmune disorders, and even in brain function and mental health conditions such as depression.
An important aspect of the human microbiome is that the bacteria that compose it have co-evolved together with human populations. Previous studies of the biological and sociological features of human social interactions suggest that natural selection has shaped social network structure and function [10-14]. While early research suggests that social relationships, especially within the household, are involved in gut microbiome transmission, the distribution of the human gut microbiome across social networks has not been studied [15-17]. Unlike our understanding of pathogens and the dynamics of infectious diseases, it is not known what role social transmission plays in the composition of the healthy microbiome. Furthermore, the link between the gut microbiome and non-infectious diseases described above implies that the spread of the gut microbiome across social networks may help explain epidemiology of obesity, cardiovascular disease, and other.
The proposed research is the first attempt to study the human gut microbiome metagenome across population-level networks of social interaction, examining the impact of the microbiome on community health. By focusing on a population in Central America, the study will also contribute to our understanding of the gut microbiome diversity in low and middle-income countries, as well as in Indigenous populations. The study aims to identify social highly-transmissible strains or mobile genes associated with either increased or decreased risk of disease, leading to better epidemiological models and novel therapeutic strategies.
Our hypothesis is that different gut bacterial species will cluster in distinct regions of social networks, and that these clusters will be associated with increased risk of elevated BMI, HbA1 and MAP, even after adjusting for known risk factors. Specifically, this would imply a statistically-significant correlation between topology measures of the village social networks and the same topology measures of co-occurrence networks of gut bacterial species and clades in the village.
Watch an interview about our work on microbiome and social networks.
- Lloyd-Price J, Mahurkar A, Rahnavard G, et al., “Strains, functions and dynamics in the expanded Human Microbiome Project,” Nature 2017; 550(7674): 61-66.
- Lloyd-Price J, Abu-Ali G, Huttenhower C, “The healthy human microbiome. Genome Medicine 2016; 8(1): 51.
- Lynch SV and Pedersen O, “The human intestinal microbiome in health and disease,” New England Journal of Medicine 2016; 375: 2369-2379.
- Wilson Tang WH, Wang Z, Levison BS, et al., “Intestinal microbial metabolism of phosphatidylcholine and cardiovascular risk,” New England Journal of Medicine 2013; 368: 1575-1584.
- Turnbaugh PJ, Hamady M, Yatsunenko T, et al., “A core gut microbiome in obese and lean twins,” Nature 2009; 457: 480-484.
- Qin J, Li Y, Cai Z, et al., “A metagenome-wide association study of gut microbiota in type 2 diabetes,” Nature 2012; 490: 55-60.
- Karlsson FH, Tremaroli V, Nookaew I, et al., “Gut metagenome in European women with normal, impaired and diabetic glucose control,” Nature 2013; 498: 99-103.
- Fujimura KE, Sitarik AR, Havstad S, et al., “Neonatal gut microbiota associates with childhood multisensitized atopy and T cell differentiation,” Nature Medicine 2016; 22: 1187-1191.
- Arrieta MC, Stiemsma LT, Dimitriu PA, et al., “Early infancy microbial and metabolic alterations affect risk of childhood asthma,” Science Translational Medicine 2015; 7: 307ra152-307ra152.
- Fowler JH, Dawes CT, and Christakis NA, “Model of genetic variation in human social networks,” Proceedings of the National Academy of Sciences 2009; 106: 1720-1724.
- Fowler JH, Settle JE, and Christakis NA, "Correlated Genotypes in Friendship Networks," Proceedings of the National Academy of Sciences 2011; 108(5): 1993-1997.
- Christakis NA and Fowler JH, “Friendship and Natural Selection,” Proceedings of the National Academy of Sciences 2014; 111: 10796-10801.
- Nishi A, Alexander MA, Fowler JH, and Christakis NA, “Assortative Mating at Loci Under Recent Natural Selection in Humans,” Bio Systems 2020; 187: 104040.
- Kim DA, Benjamin EJ, Fowler JH, and Christakis NA, “Social Connectedness Is Associated with Fibrinogen Level in a Human Social Network,” Proceedings of the Royal Society B 2016; 283: 20160958.
- Brito IL, Yilmaz S, Huang K, Xu L, Jupiter SD, Jenkins AP, Naisilisili W, Tamminen M, Smillie CS, Wortman JR, Birren BW, Xavier RJ, Blainey PC, Singh AK, Gevers D, and Alm EJ, “Mobile genes in the human microbiome are structured from global to individual scales,” Nature 2016; 535(7612): 435-439.
- Sarkar A, Harty S, Johnson KV-A, et al, “Micorbial transmission in animal social networks and the social microbiome,” Nature Ecology & Evolution 2020; 4:1020-1035.
- Brito IL, Gurry T, Zhao S, et al. “Transmission of human-associated microbiota along family and social networks,” Nature Microbiology 2019; 4:964-971.
- Shakya HB, Stafford D, Hughes DA, Keegan T, Negron R, Broome J, McKnight M, Nicoll J, Nelson JA, Iriarte E, Fowler JH, Christakis NA, “Exploiting social influence to magnify population-level behavior change in maternal and child health: A Randomized controlled trial of network targeting algorithms in rural Honduras,” BMJ Open 2016; 7:e012996.
02 Research
![]()
![]()
Brain Tumor Genomcis
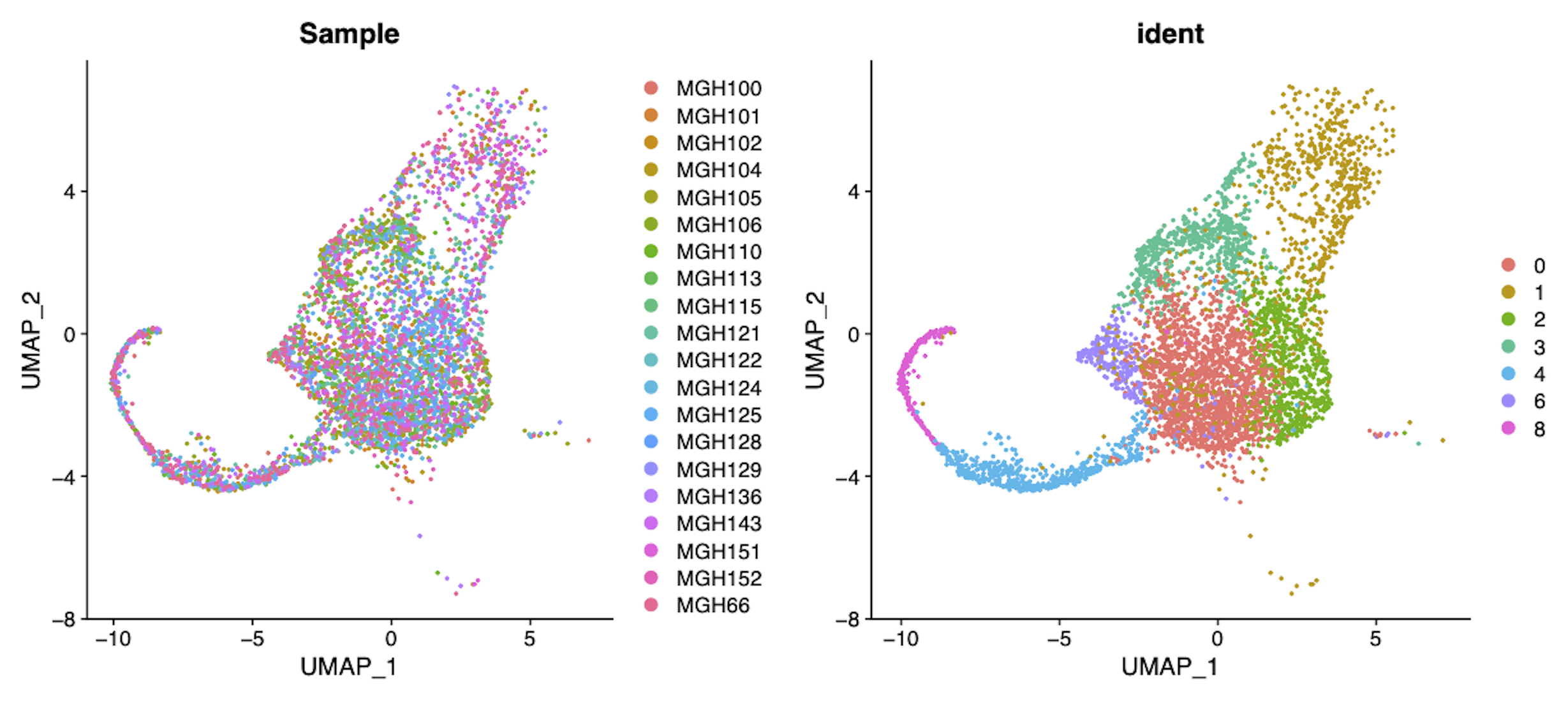
Single-cell RNA-Seq identifies 7 distinct cell types across 20 adult GBM tumors (Data from Neftel, 2019)
A study of single-cell RNA-Seq in order to identify gene signature of glioblastoma cancer stem cell, and identify potential new targets for therapy.
Single-cell multiomic profile of pituitary adenomas
Prashant Chittiboina (PI)
Prashant Chittiboina (PI)
A study of epigenomics of pituitary adenomas and normal pituitary cells to identify transcriptional regulation mechanisms responsible for pathogenesis of spontaneous pituitary adenomas.
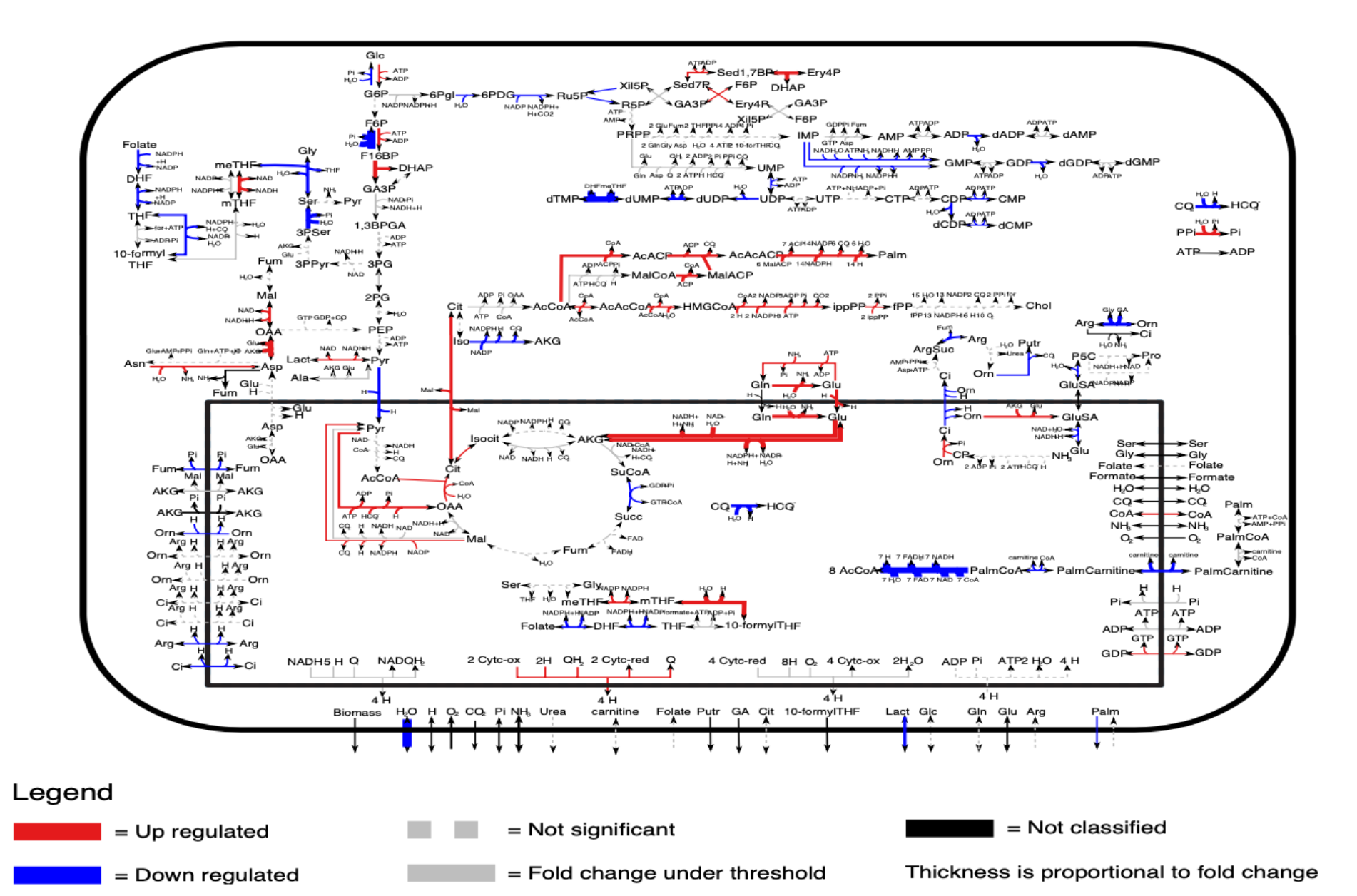
Differentially activated metabolic pathways across different tumors (Data form TCGA)
Metabolic pathways of low grade gliomas
Jack Goodman (collaborator)
Jack Goodman (collaborator)
We use metabolic pathway reactivity scores from transcriptiome sequencing data to identify low grade glioma tumors associated with prolonged patient survival.
Metablic pathway classification of tumors and prognosis of survival
Jack Goodman (collaborator)
Jack Goodman (collaborator)
We use survival modeling based on differential metabolic pathway activation to offer novel classificaiton system for tumors across all tissue types and use metabolic classification of tumors to identify patients with prolonged survival.
03 Research
![]()
Click here for more information about the Human Nature Lab work on face-to-face network interventions.
Social Networks and Health
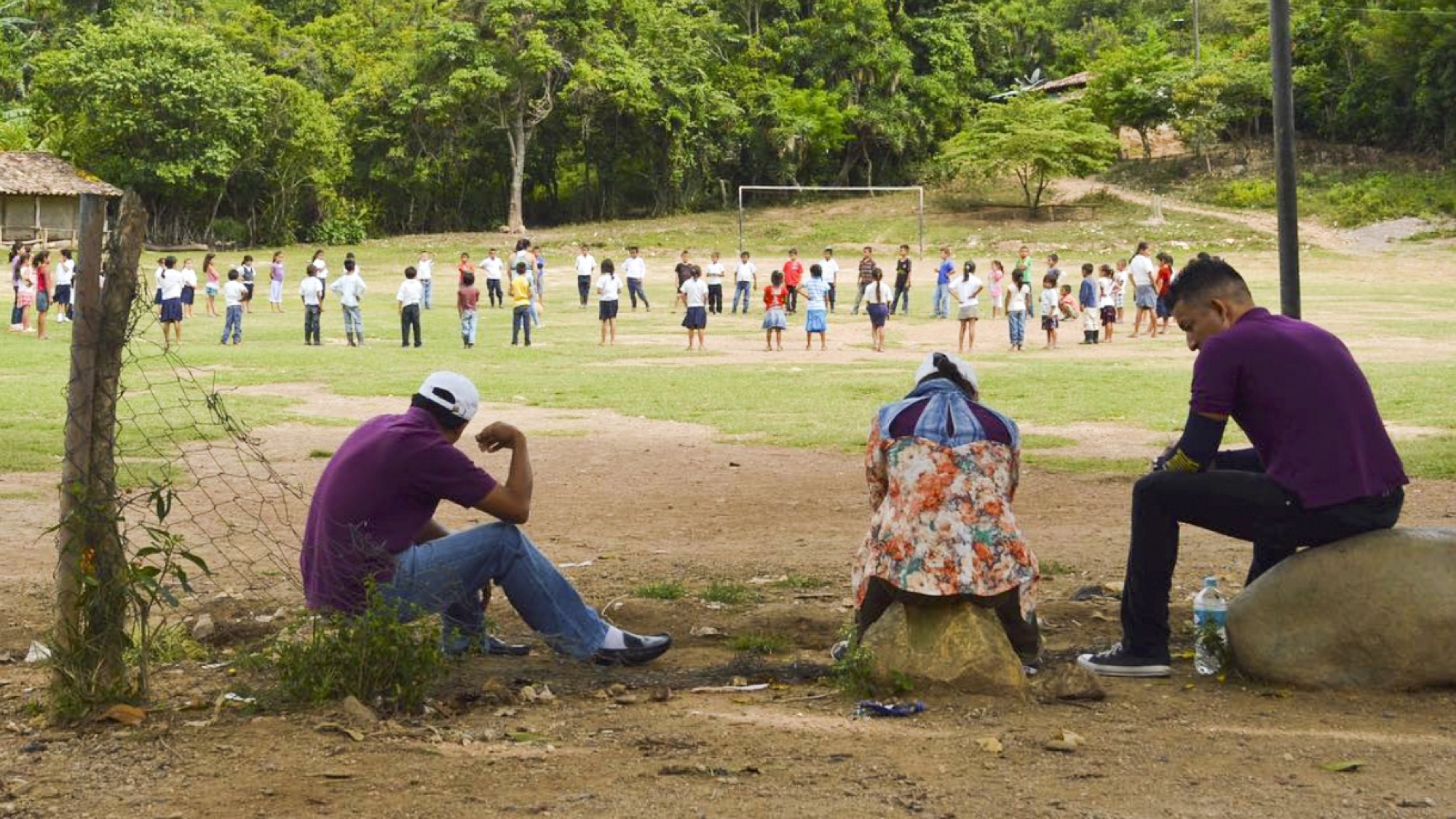
Setting of HNL RCT in network targetting to improve maternal and child health in the highlands of Honduras
Watch a video about our field work in Central America
Watch a video about our Trellis app that we use to map real-world face-to-face social networks.
Watch a video about our breadboard software platform for conducting social network experiements.
Click here for more information about the Human Nature Lab work on face-to-face network interventions.